Causal inference analyses often use existing survey data, which in many cases are clustered. In this work we discuss propensity score weighting methods in a multilevel setting where within clusters individuals share unmeasured variables that are related to treatment assignment, outcome distribution, and treatment effect. Particularly we focus on the case where multilevel modeling approaches are either not feasible or not useful due to the presence of a large number of small clusters. We found, both through numerical experiments and theoretical derivations, that the strategy of grouping clusters with similar treatment prevalence and estimating propensity scores within such cluster groups is effective in reducing bias from unmeasured cluster-level covariates. We restrict our inference population on the sample in our current study, but we expect to extend the partially pooling method in generalizing causal effect into the population by incorporating survey weights. We apply our proposed method in evaluating the effectiveness of center-based pre-school program participation on the child’s achievement at kindergarten, using the Early Childhood Longitudinal Study, Kindergarten data.
This is a joint work with Trang Nguyen and Elizabeth A. Stuart from Johns Hopkins Bloomberg School of Public Health.
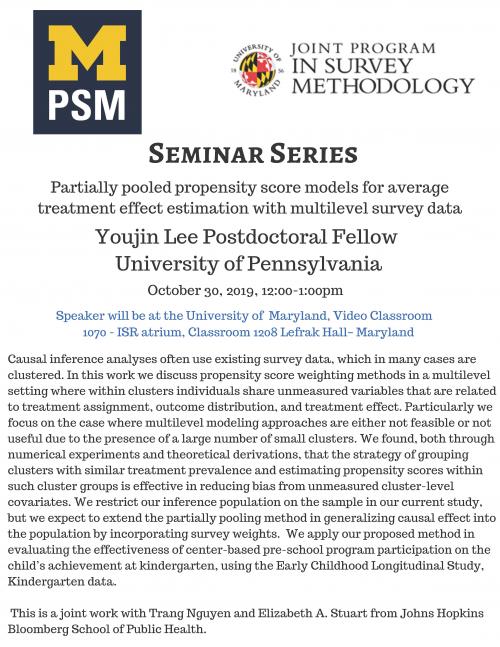